The concept of Customer Lifetime Value (CLV) might seem self-explanatory, but there are lots of misconceptions of what CLV is and how we can best use it. In short, CLV is a model that estimates the value that a customer brings to your company during its expected time of customer loyalty. If implemented using adequate analytical techniques and predictive models, a high number of use cases open up, including value-based segmentation, evaluation of marketing initiatives and optimisation of acquisition.
Customer Lifetime Value is a broad concept that spans everything from getting a one-number measurement of the total value of your customer base to being used as a prescriptive tool serving as the underlying basis for all decision making around the management of individual customers. Your company’s appropriate level of ambition is decided by different factors, including business needs, quality and availability of data, availability and the skill level of your resources, and the company’s overall strategy and vision for data-driven decisions. Regardless of your level of ambition, CLV is something that, if done correctly from the start, can be built upon either when the need or objective increases.
As the name suggests, CLV is naturally split into three different entities: customer, lifetime and value. In the simplest model, the customer entity is represented by the average customer, whereas in a richer and more useful model, it is represented by the individual customer itself. Pursuing the latter might seem too challenging for some companies, but it is a wholly necessary step to take when going from a product/service based way of working to a customer-centric approach.
When considering the customer’s lifetime, it is quite trivial that the longer a customer stays with you, the more value you have the opportunity to gain during that time. However, the lifetime of a customer is, in most cases, unknown (unless the customer has entered a time-bound contract) and would need to be estimated with predictive modelling. This part is usually hard to determine precisely and will also be one of the main uncertainties in your model.
The last entity, value, is the net gross profit that this customer is expected to bring to your company. A proper estimation of a customer’s value takes both relevant costs and revenues into consideration. A combination of analytical models and accounting can often provide a reasonable estimate of the customer’s value up until this date. This value cannot be changed or impacted in any way, but it can be useful to include in some use cases. However, a customer’s future expected value is affectable and is where one can have the most significant impact. More advanced prediction models need to be deployed to get a good estimate of the value for the rest of the customer’s lifetime.
Read Analytics Tactic Spotlight: Recommendation Engine
It is also wise to consult one of your company’s controllers to ensure correct input data and to understand the appropriate acceptance level of the predictions. After doing so, putting all of the above pieces together will give you a model which estimates how much a customer is worth to your business. Once this model is deployed, it opens up a great number of use cases for improving and optimising your business. Let’s take a look at a couple of uses cases that can bring value to your company.
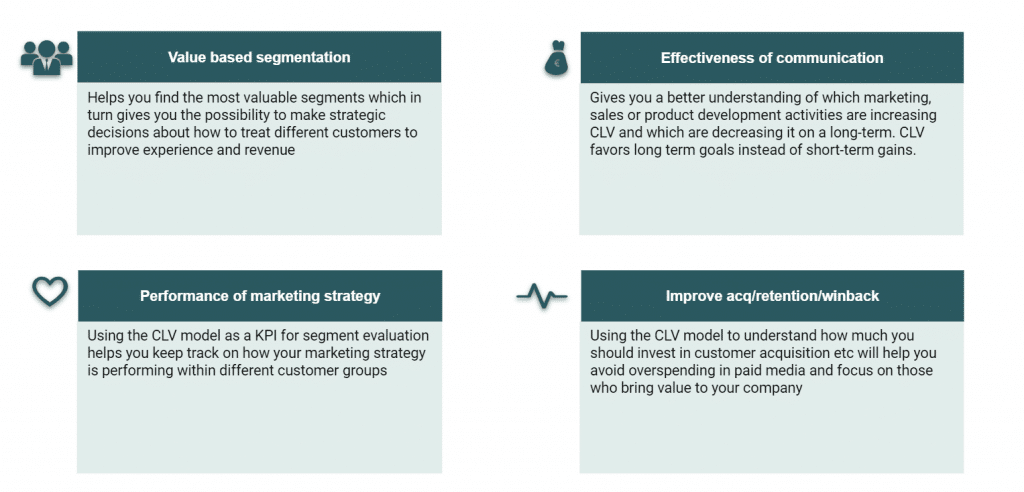
Exhibit 1: Four reasons why CLV is important to measure
Use case 1: Value-based Segmentation
One of the most common use cases of the CLV model is to perform a value-based segmentation of the customer base. While many segmentation models try to segment customers based on demographic data and/or purchase data, this segmentation aims at dividing your customer base into groups based on the value they bring to your company. One of the advantages of segmentation based on CLV is that it will account for future retention of customers, which is a common drawback for simpler segmentation models. A typical CLV segmentation will group your customer base into three groups: Low value, Mid value, High value.
Based on this segmentation, you can then take different actions depending on what segment the customer belongs to. If the customer is a low-value customer, you should probably not spend too much time, if any, dealing with this customer. The high-value customers might get access to your premium service and can skip queues to get in direct contact with customer support or a key account manager. Retention campaigns or anti-churn programs can also focus on the high-value customers to maximise their monetary effect.
Use case 2: Acquisition Optimisation
One well-tried and successful approach has been to identify potential customers that are similar to existing customers with a high CLV and then focus the acquisition to target those customers. If you know what a high-value customer looks like, you can start focusing on acquiring them instead of putting effort into acquiring low-value customers. Doing this will yield a higher return on investment on the money spent on acquisition.
It is also possible for a predictive CLV model to estimate the potential value for a customer even before they become one. Combined with an estimate of how much an increase in acquisition spend will increase actual acquisition, CLV will serve as an optimisation tool for acquisition costs. At your hand, you now have a tool for estimating at what level the acquisition spend will give you the most ROI per customer.
If you are interested in CLV, its other use cases not mentioned in this post, or how CLV can benefit your organisation, feel free to contact us.
Contact us
Written by Henrik Nordström, Sebastian Ånerud, and Eric Hörberg