This recipe describes how to build an efficient model (taxonomy) for your media buying. The model will enable you to achieve significantly more with your current media budget. Here we are going deeper into the subject, offering actionable insight into how you could structure your digital media buying efficiency.
Achieving a 25% Efficiency Gain in Media Purchasing
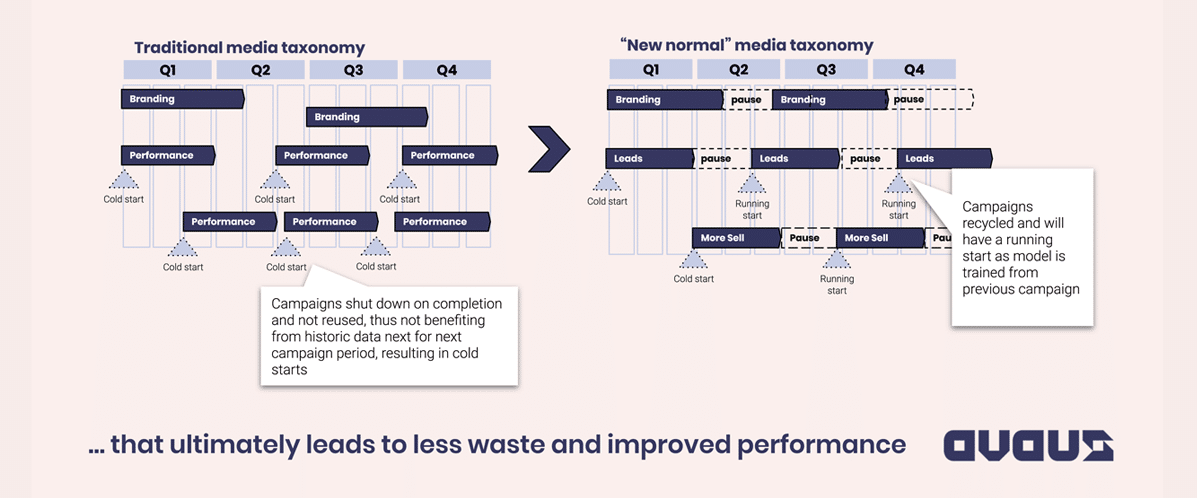
Exhibit 1: Creating a campaign structure to support Facebook’s & Google’s ML-functionality
Stop Thinking About Campaigns and Start Thinking About Digital Media as Business & Sales Processes
As walled gardens emerged and changes in how data could be gathered and activated, it has become increasingly important to work within those walled gardens. Enhance your competitiveness by leveraging a platform’s built-in functionality to its full potential.
We believe that many digital media promises are yet to be fulfilled, as many media buyers still plan and execute digital media as if it were old school media. They use media in the form of campaigns on a timesheet instead of seeing them as a business process and part of the customer journey. We fail to see the digital channels for what they are, so our decisioning does not allow them to live up to their promise.
The promises we are referring to are timely and targeted communication, fueled by automation and smartness (ML & AI).
The traditional perspective of a campaign’s planning & activation is predominantly focused on planning something around a budget and time constraint. This leads to a lack of focus on the actual business process and the kind of objectives that need to be set. Identifying your objective is crucial to your digital campaigns. As it says in the FB ads documentation:
“Before you create an ad, first consider what your business goals are. It’s important to know what you want to achieve in order to choose the right objective”.
When you set such an objective, you tell machine learning models how they should optimise. It could be brand awareness goals such as optimising for reach, consideration goals such as traffic or app installs, and, lastly, goals such as conversions or store traffic. The built-in ML model will then learn to perform on that objective with the help of the vast amount of data they have access to and the direction you’re providing with the use of first-party and/or pixel data. This process goes for most digital media channels and platforms.
First of all, decide what your model should optimise (set your objective) and secondly what direction (data) to give it. And don’t throw away your model. You will waste time and resources if you have to retrain it next time you’re doing the same kind of exercise.
Getting Started
- Assess and ensure ownership of data and algo
Start by assessing the current state of your digital media from a model, data and process perspective. Look back at past campaigns and try to map them after objective (business goal) and data (segments & direction). Campaigns that share the same idea and logic can be grouped into performance buckets. For example, being a fashion retailer, one of these buckets could be selling sneakers, selling a specific brand of sneakers or selling high-value clothing.
- Create a taxonomy
When you’ve assessed your current state, it’s time to create and implement a taxonomy built around these performance buckets. The idea is to create a structured approach that is persistent, i.e. campaigns that can be reused each time they are needed. Ensure that all campaigns are set up to fully leverage data and algorithms. Treat your digital campaigns as machine learning exercises.
- Start running tests
With your new taxonomy and campaigns set up, start testing and use in-flight optimisation. Remember, the goal is to create persistent performance buckets, so the goal should be to optimise as you go to hone in on your target.
- Recycle campaigns
When a campaign budget runs out, and the campaign is no longer needed, don’t delete the campaign model. A recurring business process may be able to use the pre-trained campaign for a running start. This will lower your media budget and accelerate the results, as campaign models will have shorter learning cycles.
Models & Data
So, to find new efficiency gains within digital marketing, we need to rethink how we plan and execute digital media. We need to shift our mindset from time & budget towards models & data. If we don’t, our decisioning and limitations will put the model’s performance at risk, which means wasted media spending. You need to put extra thought into how you structure your campaigns, ad sets and ads in a beneficial way for the buying platform’s ML models to perform.
This way of working not only helps those algorithms to perform, but its structure also enables ownership of the whole buying process, easier implementation of automation to further increase efficiency and a much better environment for experimentation.
If this feels daunting, you could always start with a proof of concept. Re-use a previous campaign that shares the same objective and target audiences, just put in the new creatives and the budget. See what improvements you can find, how quickly the campaign gets up to speed and what the end results are.
If you need help with these steps, ask your media agency partner, or use Avaus as a mediator!
Contact us